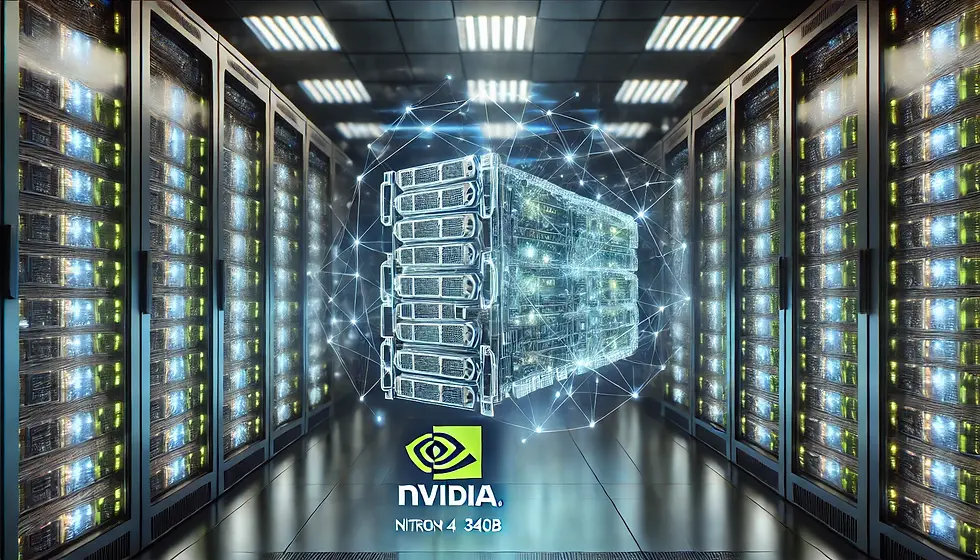
NVIDIA has once again pushed the boundaries with the release of their latest model, the Nitron 4 340B. This open-source behemoth, boasting 340 billion parameters, is specifically designed to generate synthetic data for training smaller models. This development holds significant promise for the open-source community, where access to high-quality datasets is often a formidable challenge.
Synthetic data has emerged as a crucial resource in AI training. It provides the means to create diverse and high-quality datasets that can enhance the performance, accuracy, and robustness of machine learning models. Traditionally, acquiring such datasets is an expensive and complex process, often involving extensive data collection and processing. NVIDIA’s Nitron 4 340B offers a groundbreaking solution by enabling developers to generate these datasets freely and at scale.
Nitron 4 340B is optimized to work seamlessly with NVIDIA’s ecosystem, including Nemo and Tensor RT. It is part of a family of models that include base, instruct, and reward models, forming a comprehensive pipeline for synthetic data generation. The model’s architecture is tailored to mimic the characteristics of real-world data, thereby improving the quality and relevance of the generated data.
Developers can access Nitron 4 340B through multiple platforms, including NVIDIA’s own AI portal and Hugging Face. The model is packaged as a microservice, making it easy to deploy and integrate into various workflows. This accessibility is a boon for startups and smaller teams that lack the resources to generate high-quality data independently.
The performance of Nitron 4 340B has been rigorously tested using a variety of benchmarks and rubrics. For instance, in generating Python scripts, the model demonstrated efficiency and accuracy comparable to established models like GPT-4. However, it showed limitations in more complex tasks, such as coding challenges and logical reasoning problems. Despite these setbacks, the model excelled in generating coherent and contextually appropriate responses in many scenarios.
One of the standout features of Nitron 4 340B is its reward model, which filters responses based on attributes like helpfulness, correctness, coherence, complexity, and verbosity. This ensures that the synthetic data generated is of high quality, making it an invaluable tool for training custom LLMs.
The implications of Nitron 4 340B for the AI community are profound. By providing a scalable and free method for generating synthetic data, NVIDIA is democratizing access to resources that were previously the domain of well-funded organizations. This has the potential to accelerate innovation and development across various AI applications, from natural language processing to computer vision.
Moreover, the open-source nature of Nitron 4 340B encourages collaboration and continuous improvement. Researchers can customize the model using their proprietary data, enhancing its applicability and performance across different domains. As more developers and researchers adopt and contribute to the model, its capabilities are expected to grow, driving further advancements in AI technology.
NVIDIA’s Nitron 4 340B represents a significant leap forward in synthetic data generation. While it may not be perfect, its strengths far outweigh its weaknesses, offering a robust and accessible tool for the AI community. As synthetic data becomes increasingly vital for AI training, innovations like Nitron 4 340B will play a crucial role in shaping the future of artificial intelligence.
#NVIDIA #AI #SyntheticData #MachineLearning #OpenSource #Nitron4 #DataGeneration #ArtificialIntelligence #ModelTraining #AIDevelopment
Comentarios