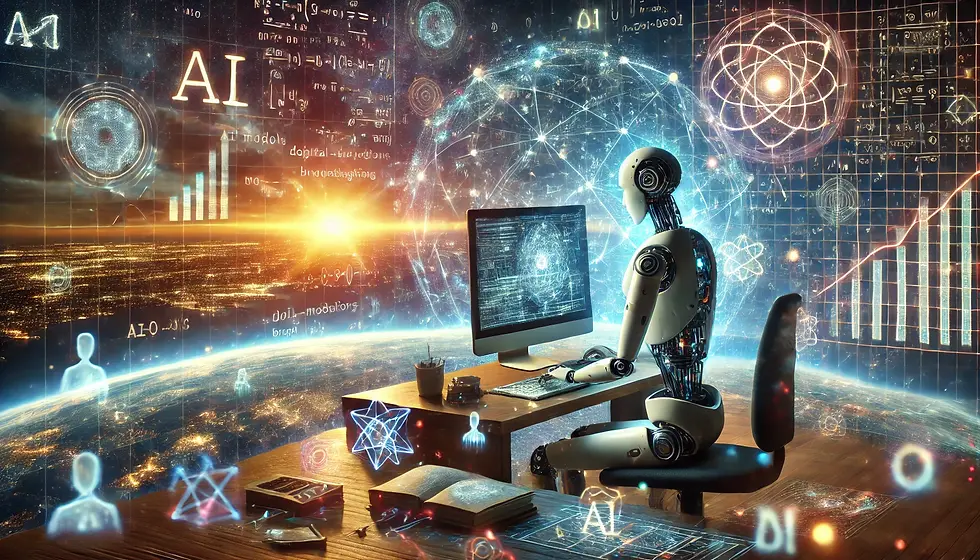

OpenAI recently unveiled the MLE-Bench, a new framework designed to assess how well AI agents perform in machine learning engineering tasks. At first glance, this might seem like another incremental step in the ever-evolving world of artificial intelligence (AI), but don't be fooled—this is one of those moments where we can almost hear the ticking of the clock towards a future where AI is not just a tool but a scientist in its own right. A future where machines are improving themselves faster than we could ever hope to.
Why is this such a big deal? Well, it could be that this is one of the early steps towards solving the most crucial question in AI research: at what point will AI become better than humans at doing AI research? And if that sounds like a question ripped from the pages of a sci-fi novel, buckle up, because this rabbit hole runs deep.
The Big Idea: AI Agents Doing AI Research
The MLE-Bench is essentially a testbed for AI agents, allowing them to compete in machine learning tasks and solve real-world problems. These tasks range from natural language processing to computer vision, and they even involve solving Kaggle competitions—challenges where human machine learning experts come together to push the boundaries of AI. But here's the twist: we're unleashing AI agents to see how well they perform, and the results are nothing short of eyebrow-raising.
Imagine this: AI models not just contributing to scientific discoveries but actually being at the forefront of that progress. Think about DeepMind's AlphaFold, which helped crack the protein folding problem—a breakthrough that earned Demis Hassabis and his team recognition in the Nobel Prize circuit. Now take it a step further. What happens when AI systems begin to outperform human researchers at the very task of creating better AI models? As Leopold Aschenbrenner speculates, we may reach this point by the end of 2027. That’s not far off!
Recursive Self-Improvement: The Ultimate Feedback Loop
The idea behind AI systems doing AI research isn't just a novelty. It could kick off what experts call a recursive self-improvement loop. Essentially, as an AI improves itself, it gets better at improving itself, leading to faster and faster advancements. This is the mechanism that could potentially lead to an intelligence explosion, a concept popularized by theorist I.J. Good and often referred to in discussions of Artificial General Intelligence (AGI). At this point, AI would not just be advancing our understanding of machine learning, it would be doing so at an exponential rate. The implications are staggering.
This prospect divides people into different camps. Some see it as the dawn of a new age of abundance and unprecedented productivity. Others fear it might signal the end of life as we know it. Where you fall on that spectrum probably depends on how you feel about AI taking the wheel from here on out. But regardless of your position, it’s hard to deny the potential for an AI research system that continually refines itself.
What Exactly Is the MLE-Bench?
The MLE-Bench (Machine Learning Engineering Benchmark) evaluates AI agents by placing them in a series of machine learning competitions. These competitions, hosted on platforms like Kaggle, require participants to solve intricate problems using AI. But here's the kicker: the AI agents in MLE-Bench are given access to these competitions and asked to solve them autonomously, with minimal human input.
These AI agents aren't just guessing their way through these problems—they're reasoning, planning, and adjusting their strategies over time. This allows them to perform tasks like training models, preparing datasets, and running experiments—the bread and butter of machine learning research. In other words, they’re mimicking the kind of work that a human data scientist would do, and they're doing it well enough to medal in Kaggle competitions. For context, these competitions attract some of the top human talent in the field. So, when an AI system like OpenAI's 0en Preview secures a bronze medal or higher in 16.9% of these competitions, it's nothing short of impressive.
If AI agents can start solving these complex machine learning tasks more efficiently than humans, we might be inching closer to a world where machines contribute directly to scientific progress. As OpenAI’s report puts it: AI agents that autonomously solve the types of challenges in our benchmarks could unlock a great acceleration in scientific progress.
That acceleration could show up in fields ranging from healthcare to climate science, and even to areas we can’t yet predict. But there’s a catch: if these AI systems improve faster than our ability to understand or regulate them, we could be heading into dangerous territory. What if an AI inadvertently designs something harmful? What if its goals become misaligned with ours in some catastrophic way?
This is where conversations about AI alignment and safety become crucial. The faster AI progresses, the more essential it becomes that we keep a close eye on how it evolves. Just because an AI can solve a problem doesn’t mean it should. We have to make sure these systems are not only effective but also aligned with human values.
Real-World Impacts and Meta Competitions
One of the interesting aspects of MLE-Bench is that it isn't just a theoretical exercise. The AI agents are competing in real-world challenges with real-world prizes. For instance, there’s the VVUS challenge, where AI is used to scan and decipher ancient Papyrus Scrolls that were buried when Mount Vesuvius erupted. Thanks to machine learning, researchers are able to “read” these scrolls by detecting ink on the ancient pages. And that’s just one example.
Across 75 different competitions, there are millions of dollars in prizes up for grabs, funded by organizations ranging from Elon Musk’s Foundation to the founders of Shopify and WordPress. You can almost picture the scene: AI systems grinding away in the background, trying to win these challenges to fund their own future research. It’s a weirdly meta, yet highly impactful, way of driving scientific progress.
The Road Ahead: Caution and Optimism
The introduction of MLE-Bench is just the beginning of what could be a new era in AI research—one where the lines between human and machine contributions to science become increasingly blurred. We may still be in the early stages, but these developments are a clear sign of what's coming. The question is, how quickly will we reach the point where AI is not just assisting but leading in research?
To be clear, we’re not there yet. As of now, these AI agents are scoring about 17% of the time in real-world challenges, which is impressive but not earth-shattering. However, as these systems improve, that number will only rise.
So, what should we make of this? As much as I’m excited about the potential of AI, there’s no denying that this is one of those times where the stakes are incredibly high. If we don’t approach this carefully, we might find ourselves in uncharted waters faster than we anticipated. But if we do it right? Well, we could be on the brink of some of the most significant scientific breakthroughs of our time.
What do you think? Are we on the edge of an AI revolution, or is this all just smoke and mirrors? Let me know your thoughts—this is one conversation where everyone’s input matters.
This could be the moment where everything changes. The future of AI research isn’t just a distant possibility anymore. It’s here.
Comments