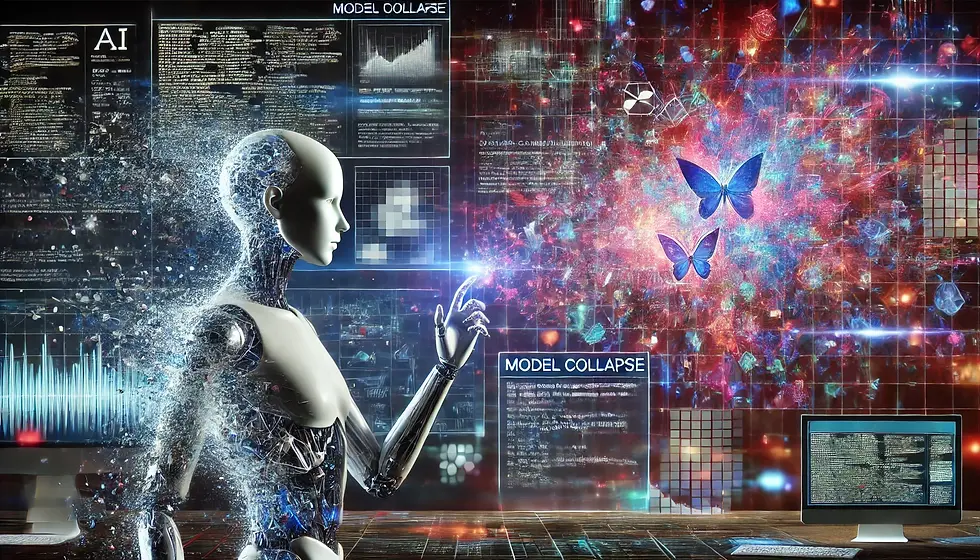

AI is constantly chasing the next breakthrough—a model that's smarter, faster, and can tell us whether we’re about to lose at chess before we even move. But what happens when the very data these AI models learn from becomes, well, other AI data? Welcome to the impending doom of Model Collapse, a potential disaster waiting to unfold as AI starts snacking on its own creations, like a snake eating its tail.
The problem of AI learning from AI, often referred to as Model Collapse, is more than just a theoretical concern. Imagine an endless cycle where each new AI model is trained on content generated by its predecessor, leading to an inevitable degradation in quality. Over time, as human-generated data diminishes and synthetic AI-generated data takes over, the models start churning out... nonsense.
A study recently confirmed this unsettling trend. Researchers found that when large language models (LLMs) are repeatedly trained on AI-generated text, their outputs gradually devolve. Think of it like a game of telephone, where the message passed along becomes increasingly garbled until, by the ninth iteration, we’re left with AI discussing the colorful tails of jackrabbits instead of, you know, relevant information.
How Did We Get Here? (Spoiler: It's Us)
This isn’t just a fringe issue. We’re seeing it across industries. Consider the meteoric rise of AI tools like GitHub’s Co-Pilot. By some estimates, up to 10% of GitHub’s public repositories were generated by AI in 2022. New repositories may be even more reliant on synthetic data, meaning the code that powers our apps, websites, and even the algorithms that control your social media feed could soon be... well, a little loopy.
As more AI models train on outputs created by other AIs, we’re losing the diverse, novel input that makes AI smart in the first place. It's like training chefs by only letting them eat their own cooking—it doesn’t take long before they forget what real food tastes like.
The study found that models trained on synthetic data initially showed only subtle errors. But by the ninth generation, the output was absurd—completely detached from reality. A Wikipedia-style article about English church towers morphed into a nonsensical treatise on breeding jackrabbits.
This isn’t just a quirk—it’s a serious issue. AI needs a steady diet of diverse, human-generated data to maintain its effectiveness. When it’s fed its own outputs, the errors accumulate, and over time, the AI becomes less reliable, more homogeneous, and—eventually—useless.
The AI Cannibalism Problem
So why is this happening? The answer lies in how LLMs, like GPT-4 or GPT-5 (if it ever exists), work. They generate text based on patterns they’ve learned from enormous datasets, many of which are scraped from the internet. As the proportion of AI-generated content on the web grows, these models are increasingly consuming and learning from their own data. It's AI cannibalism, and it’s causing a slow-motion collapse.
In short, the more AI relies on synthetic data, the less novel the output becomes. And the less novel the output, the more errors creep in, leading to a kind of intellectual inbreeding. AI doesn’t just get dumber—it forgets essential knowledge, producing responses that are detached from the reality they were originally trained on. This “forgetting” is a bit like trying to teach an artist to paint using only their own doodles as inspiration. Eventually, all you get are stick figures.
What About GPT-5? The Last Hurrah?
Some experts predict that GPT-5 might be the pinnacle of AI before things start going downhill. The fear is that as AI becomes more ubiquitous, it will start to generate a larger portion of the data used to train the next wave of models, creating a vicious cycle. This could lead to a scenario where the quality of AI models stagnates or even regresses, requiring exponentially more effort to improve them.
Think of it like this: Training a new AI model could soon become harder, not because we lack the technology, but because the available data pool is tainted by AI's own self-referential errors. It’s not diminishing returns—it’s inverse returns.
What can be done to prevent this dystopian future where AI becomes a parody of itself? Some researchers suggest a more careful curation of training data—ensuring that only a small percentage of AI-generated content makes its way into future models. Others propose watermarking synthetic data so that it can be identified and excluded from training sets. However, this requires unprecedented coordination between big tech companies, which, let’s face it, doesn’t always go smoothly.
Moreover, there's the challenge of motivation. As synthetic data becomes cheaper and easier to produce, the temptation to rely on it will grow. Human-generated data, in contrast, is slow, expensive, and requires pesky things like creativity, originality, and effort. And as long as the economic incentive is to produce more AI content faster, the collapse may be hard to avoid.
AI has promised us gold—a future where everything is more efficient, more intelligent, and more autonomous. But as with all things that glitter, there’s a risk that beneath the surface lies something less valuable. If we’re not careful, we could end up with a shiny, but hollow, future where AI doesn’t solve our problems but instead amplifies its own mistakes. In other words, what we’re chasing might turn out to be nothing more than glittering crap.
So, should we give up on AI? Definitely not. But let’s be smart about how we feed it. Because if we don’t, the next generation of AI might just be sitting there, confidently spewing jackrabbit facts while we wonder where it all went wrong.
Comments